A commenter on Why The Law wondered whether we can "have a society whose output has equivalent K-complexity as ours, but is generated from simpler rules"—in effect seeking the simplest legal system that can sustain something like the U.S. economy.
The idea reminded me of two papers—"Economic Production as Chemistry," by Padgett, Lee, and Collier (2003), and "Evolution of Cooperative Problem-Solving in an Artificial Economy," by Baum and Durdanovic (2000)—because in each, the authors are trying to develop a minimal model of fruitful markets: what are the necessary conditions for productive collaboration? Which treatments of property and compensation generate stability, and which degrade? Must agents be intelligent? Altruistic?
Hypercycles
Padgett et al. imagine a grid-world with three basic components: firms, one in each cell, each of which has a random initial endowment of rules, which are simple procedures for transforming products. So for example the cell at (2, 3) may have a rule C -> F that takes the product C as input from one neighboring firm and spits out an F to another. It looks something like this:
A natural question to ask of such a world is whether it can sustain chain reactions, or coincidences where firm after firm spits out the very product that the next guy needs as input, as when a firm with rule A -> B happens to send off its B to a firm with rule B -> C.
In fact the model is deliberately set up with these chains in mind. Here are the rules of the game:
- Initially, technologies are distributed randomly among the firms, which are arrayed on a large grid with wraparound—so that every firm, even if it's on an "edge," has eight potential trading partners.
- In each "round" of the model (which proceeds in asynchronous discrete steps):
- A random rule is chosen or "activated."
- The firm that owns this rule grabs a product from its "input environment," modeled as an urn containing a bunch of products.
- If the product it chooses is an input for the activated rule (e.g., if the product is a C and the rule is C -> Z), the rule does its thing and the output of its transformation is passed randomly onto one of the firm's neighboring trading partners.
- Otherwise, the input product is sent to an output environment (also modeled as an urn).
- The round's action continues as firms receive their neighbors' output. This step is important: if you've just successfully transformed A into Z, and pass the Z onto me, and I have a Z -> V rule, then we've just engaged in a "successful transaction."
- Every time there is a successful transaction, one of the rules involved (in our example above, that would be one of A -> Z or Z -> V) is reproduced. It turns out that the behavior of the overall model depends a great deal on which one of the source or destination rules is copied. (See below.)
- To encourage competition, every time one rule is reproduced, another one, chosen randomly, is killed off. So the total number of rules in play is held constant. This selection pressure, combined with the ability for rules to reproduce, is what keeps the world from degrading into an unproductive mess. (Again, more on this below.)
- Firms that run out of rules die or "go bankrupt." Products sent their way are immediately directed to the output environment.
- Firms continue to pass products around until one of these lands on a firm that doesn't have any compatible rules (i.e., if a D shows up at a firm that only has Z -> F, E -> F, and C -> D). At that point, the product is ejected into the output urn and a new rule is randomly chosen, as in step 1. (Meanwhile, and here's the "asynchronous" part, other firms are passing around other products. So the whole model doesn't reset when a single chain comes to an end.)
So how does anything interesting happen in this world? How do firms even survive? The short answer is "loops":
In this model, the minimal requirement for long-term survival, both of firms and of clusters, is to participate in at least one spatially distributed production chain that closes in on itself, to form a loop. Not all production chains within a trading cluster need be closed into loops. And more than one loop within a cluster is possible, in which case we may have a dense hypercyclic network of spatially distributed production rules. But loops within distributed chains of skill are crucial, not for production itself, but for the competitive reproduction of skills. Loops set up positive feedbacks of growth in skills that give firms that participate in them the reproductive ability to out-produce firms that do not. Put another way, clusters of firms can produce products with or without hypercycles, but firms whose skill sets participate in production chains that include loops have the further capacity to keep renewing each other through time. This is the chemical definition of life.
So the key is to promote these stable hypercycles. And it turns out that for loop maintenance, the model's most important ingredient is one touched on in step #4 above—the choice between "source" and "target" reproduction:
In the spatial topology setting, there are two variants of “learning by doing” that can and will be explored:
- “Source reproduction” is where the originating rule in a successful transaction is reproduced.
- “Target reproduction” is where the receiving rule in a successful transaction is reproduced.
For example, if A -> B receives an A from the input environment, transforms it into a B, and then successfully passes that B onto a neighboring B -> C, who transforms it again, then “source reproduction” is where the initiating A -> B reproduces, and “target reproduction” is where the recipient B -> C reproduces. Variation in mode of reproduction thus defines who benefits from the transaction.
We think of source reproduction as “selfish learning,” because the initiator of the successful transaction reaps the reward (like a teacher). And we think of target reproduction as “altruistic learning,” because the recipient of the successful transaction reaps the reward (like a student).
So which works better?
...in comparison with source reproduction, target reproduction dramatically increases the likelihood of growing stable hypercycles. And it also increases the spatial extensiveness and complexity of the firm cluster that hypercycles produce.
And why?
As explained in Padgett (1997), the basic reason for this superiority of target reproduction, or altruistic learning, is repair. Target reproduction combats dynamic instability in a way that source reproduction does not. The basic process of dynamic instability, causing hypercycles to crash, is that as one skill reproduces rapidly, under competition other skills are driven to zero, thereby breaking the reproductive loop of skills. Spatial topology distributes this dynamic into an overlapping series of neighborhoods, thereby inducing local heterogeneity. This opens the door for localized co-adaptive feedbacks to operate. But source reproduction, or selfish learning, does not really attack the basic dynamic instability itself. Source reproduction is this: an initial activated rule passes on its transformed product to a neighboring compatible rule, causing the original activated rule to reproduce. Under source reproduction, frequently activated rules reproduce more frequently, often eventually driving out of business even compatible neighbors on whom they depend for their own survival. As we shall see in the next section, other factors like endogenous environment can sometimes moderate this destructive dynamic, but source reproduction in and of itself does not eliminate the non-spatial instability problem.
In contrast, target reproduction is this: an initial activated rule passes on its transformed product to a neighboring compatible rule, causing the recipient rule to reproduce. Here the more frequently the initial rule is activated the more frequently the second recipient rule reproduces. In this way, a hypercycle can repair itself: as the volume of one skill in a loop gets low, high volumes of compatible skills in neighboring firms reach in to that low-volume skill to build it back up. Peaks and valleys along loops are smoothed.
To help get a grip on this distinction, it's worth asking whether there are any real-world examples of "altruistic reproduction." These would be domains in which the output of "upstream" firms promotes the growth of other firms down the line, thereby creating a rich self-sustaining production chain.
This seems true of many industries but especially true of information technology, where small software companies create huge markets (for themselves and others) by developing cheap, portable code for large enterprises. Even open source components—like Linux or MySQL—accelerate industry growth and feed opportunities back to software providers in the form of support contracts and custom coding projects. The result is symbiotic.
(In contrast, one could imagine how—in the kind of closed world explored in this paper—the success of something like a casino would have the perverse effect of draining its own lifeblood, by siphoning the bankrolls of active gamblers. In that way it could be construed as an example of "selfish (source) reproduction.")
The Hayek Machine
The immediate goal of Baum et al. is to find a way to solve computational problems with large state spaces, like a Rubik's cube. Their approach is to kickstart an economy of modules—little bits of computer code—that dynamically combine into complicated chains. If these chains were somehow arranged to exploit the structure of the problem at hand, the system would be able to limit its search to a tiny fraction of the space of possible moves.
Intelligent computation of that sort is easier said than done, and so most of the paper is devoted to exploring precisely which features of the module "economy" improve performance, and why.
Before getting into that, though, it might help to explore the simplest of the three problems under attack, called "Blocks World." With that under our belt it should be easier to understand the model more generally.
An instance of Blocks World has four stacks (0, 1, 2, and 3) of colored blocks. The leftmost stack (0) is your template—you never move any of its blocks as you play. Instead, your goal is to rearrange the blocks in the other three stacks—with the important caveats that (a) you can only grab the topmost block off a given stack and (b) you can only pick one up at a time—until stack 1 matches the template.
Here's a picture:
The "Hayek" referred to in the caption is the name of the economics-inspired computer program developed in the paper. It is of course an homage to the great Austrian economist Friedrich von Hayek, and it works as follows:
- The program is a collection of modules, or mini computer programs, each with an associated "wealth." The authors think of these modules as agents because they can interact with the world (the game).
- "The system acts in a series of auctions. In each auction, each agent simulates the execution of its program on the current world and returns a nonnegative number. This number can be thought of as the agent's estimate of the value of the state its execution would reach. The agent bids an amount equal to the minimum of its wealth and the returned number. The solver with the highest bid wins the auction. It pays this bid to the winner of the previous auction, executes its actions on the current world, and collects any reward paid by the world, as well as the winning bid in the subsequent auction. Evolutionary pressure pushes agents to reach highly valued states and to bid accurately, lest they be outbid." (You might want to re-read the preceding paragraph a few times, until you get a handle on exactly how the auctions work. It's not complicated—it's just that there's a lot of procedure packed into a few tight sentences.)
"In each auction, each agent that has wealth more than a fixed sum 10Winit creates a new agent that is a mutation of itself. Like an investor, the creator endows its child with initial wealth Winit and takes a share of the child's profit. Typically we run with each agent paying one-tenth of its profit plus a small constant sum to its creator, but performance is little affected if this share is anywhere between 0 and .25. Each agent also pays resource tax proportional to the number of instructions it executes. This forces agent evolution to be sensitive to computational cost. Agents are removed if, and only if, their wealth falls below their initial capital, with any remaining wealth returned to their creator. Thus, the number of agents in the system varies, with agents remaining as long as they have been profitable.
"This structure of payments and capital allocations is based on simple principles (Baum, 1998). The system is set up so that everything is owned by
some agent, interagent transactions are voluntary, and money is conserved in interagent transactions (i.e., what one pays, another receives) (Miller &
Drexler, 1988). Under those conditions, if the agents are rational in that they choose to make only profitable transactions, a new agent can earn money
only by increasing total payment to the system from the world. But irrational agents are exploited and go broke. In the limit, the only agents that survive
are those that collaborate with others to extract money from the world.
"When not everything is owned, or money is not conserved, or property rights are not enforced, agents can earn money while harming the system, even if other agents maximize their profits. The overall problem, then, cannot be factored because a local optimum of the system will not be a local optimum of the individual agents" [emphasis in original].
What happens when this system is let loose on Blocks World?
The population contains 1000 or more agents, each of which bids according to a complex S-expression that can be understood, using Maple, to be effectively equal to A * NumCorrect + B, where A and B are complex S-expressions that vary across agents but evaluate, approximately, to constants. The agents come in three recognizable types. A few, which we call "cleaners," unstack several blocks from stack 1, stacking them elsewhere, and have a positive constant B. The vast majority (about 1000), which we call "stackers," have similar positive A values to each other, small or negative B, and shuffle blocks around on stacks 2 and 3, and stack several blocks on stack 1. "Closers" bid similarly to stackers but with a slightly more positive B, and say Done.
At the beginning of each instance, blocks are stacked randomly. Thus, stack 1 contains about n/3 blocks, and one of its lower blocks is incorrect. All agents bid low since NumCorrect is small, and a cleaner whose B is positive thus wins the auction and clears some blocks. This repeats for several auctions until the incorrect blocks are cleared. Then a stacker typically wins the next auction. Since there are hundreds of stackers, each exploring a different stacking, usually at least one succeeds in adding correct blocks. Since bids are proportional to NumCorrect, the stacker that most increases NumCorrect wins the auction. This repeats until all blocks are correctly stacked on stack 1. Then a closer wins, either because of its higher B or because all other agents act to decrease the number of blocks on stack 1 and thereby reduce NumCorrect. The instance ends successfully when this closer says Done. A schematic of this procedure is shown in Figure 3 [below].
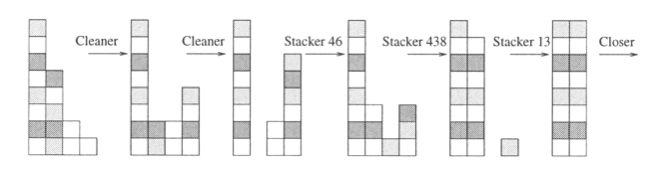
This NumCorrect that they keep referring to is a hand-coded module that counts the number of blocks in stack 1 (contiguous from the bottom up) that match the template. It's a good proxy for performance, and the measure is critical in helping the agents to know whether they're contributing value to the world (i.e., whether the program is on its way toward a solution).
If NumCorrect is left out of the system, then, performance degrades. Although it's possible for Hayek to evolve its own quick-and-dirty version, with this mere approximation it's only able to solve instances about 1/4 of the size as when NumCorrect comes hand-coded.
It's worth asking, then: how does code, be it NumCorrect or something simpler like Grab(i,j), "evolve" in the first place?
Agents are composed of S-expressions, or recursively-built trees of procedures. You can think of these as sort of like recipes:
The difference here, of course, is that whereas in the Recipe World you might have "sautée" or "chop," in Blocks World you have things like "grab" or "drop" or "look":
Our S-expressions are built out of constants, arithmetic functions, conditional tests, loop controls, and four interface functions: Look(i,j), which returns the color of the block at location i, j., Grab(i) and Drop(i), which act on stack i; and Done, which ends the instance. Some experiments also contain the function NumCorrect, and some contain a random node R(i, j), which simply executes branch i with probability 1/2 and j with probability 1/2. [This last randomizer function has the effect of "smoothing" the fitness landscape.]
All our expressions are typed, taking either integer, void, color, or boolean values. All operations respect types so that colors are never compared to integers, for example. The use of typing semantically constrains mutations and thus improves the likelihood of randomly generating meaningful and useful expressions.
It's hard to overstate how cool this process is. Out of a soup of random S-expressions—primitive codelets like adders and subtracters, if...then statements, loops, and "look" / "grab" / "drop" modules—it's possible for coherent strategical "agents" to evolve. On its own this idea is incredibly powerful: one can imagine all sorts of programs being developed automatically in this way, growing increasingly powerful by mutating and recombining useful snippets of code. Indeed, there's a whole field devoted to this approach to programming.
But what's even more spectacular is that these evolved computational lifeforms (of a sort) cooperate—via the auction process—in long computational chains, even when this requires that the initiating agents defer their rewards.
This last point is critical. It's not always obvious what the game's next best move may be, and often moves that are in the interest of long-term progress are (or look) counterproductive in the short term—as when you throw a bunch of incorrect blocks onto stack 1 to fish out an important block on stack 2. So it becomes basically impossible to solve any but the easiest Blocks World instances without the ability to "delay gratification," that is, to link up lots of low-or-no-reward-actions into chains that collectively work toward some bigger reward at their end.
The only way for this to work is to enforce the rules specified earlier:
A program cannot hope to form long chains of agents unless conservation of money is imposed and property rights are enforced. To evolve a long chain, where the final agent achieves something in the world and is paid by the world, reward has to be pushed back up the long chain so that the first agents are compensated as well. But pushing money up a long chain will not succeed if money is being created or is leaking, or if agents can steal money or somehow act to exploit other agents. If money is leaking, the early agents will go broke. If money is being created or being stolen, the system will evolve in directions to exploit this rather than creating deep chains to solve the externally posed problems. Solving the world's problems is very hard, and an evolutionary program will always discover any loophole to earn money more simply. [Eric Baum, What is Thought, p. 246.]
That's also why it's so important for auction winners in this round to pay their bids to winners from the last round. That way, those agents who most help folks downstream are able to collect larger bid payments—which helps them reproduce and stay alive—and, if their work consistently leads to large rewards down the line, their downstream partners will also reproduce (having collected money from the world) and set up yet more collaboration. It's exactly the same principle that we saw in the hypercycles world.
Conclusion
To return to the comment that kicked off this whole discussion, it now seems clearer what sorts of laws one minimally needs to sustain a rich economy. The trick is to encourage long, dynamically stable collaborative chains, and to do so requires mechanisms for transferring the rewards of productive activity to everyone who contributed; otherwise, agents who make the kind of short-term sacrifices that fuel deep cooperation will die out, and only the shallowest computation (i.e., production) will be possible.
In the hypercycles model, this was achieved by connecting reproductive success to successful transactions. Target reproduction in particular ensures that successful firms don't "burn out" their own partners.
And in the Hayek machine, property rights and conservation of money ensure that (a) the only way to earn money oneself is to create wealth for the world and that (b) money trickles back along productive chains to every contributor. [1]
These, then, are the deep compuchemical reasons that such a substantial portion of the U.S. legal system is devoted to enforcing contracts and protecting property rights. Such laws are the bedrock of our—or any—economy.
Notes
[1] Although it may seem that in these models payments go in opposite directions, they actually don't—even under a regime of "target" reproduction, hypercycles loop back on themselves, which means that A's altruistic contribution to B's reproductive success ends up helping A in the end, precisely because the two are wound up in a circle. Target reproduction works better because it keeps such circles alive, and thus allows payments to continue flowing appropriately.